Protecting areas of interest and streamlining access with Augmented Vision
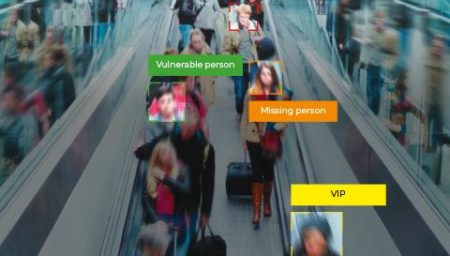
Artificial Intelligence (AI) has a very real impact on contemporary society, and we’ve only just begun to reap the benefits—and tackle the associated challenges. AI technology use cases have become more and more mainstream as deep learning algorithms help us to solve increasingly complex problems. Applied to the fields of identity and security, these technologies have driven massive progress over the last decade—progress that would not have been possible without human supervision.
Let’s be clear about one important distinction: AI allows a machine to learn on its own, but it cannot train a machine to think on its own. So what exactly do we mean by intelligence? If we go back to its Latin root, intelligence is the ability to understand—an ability that is unique to humans and inseparable from their capacity to think. As unsettling as the performance of certain recent AI applications may be, we should be careful not to jump to the conclusion that human intelligence and artificial intelligence are one and the same.
The term ‘artificial intelligence’ dates back to the 1950s, when experts were captivated by the rapid progress of computers. For decades, what passed for ‘intelligent machines’ were in fact elaborate computer programs that enabled computers to perform pre-programmed tasks, such as playing chess. Although they were groundbreaking at the time, these computers were still far from being sources of true intelligence—or understanding.
Today, AI technologies learn by trawling through reams of data and drawing conclusions based on recurring patterns. In this way, they provide humans with insights but they still lack the ability to think – and understand – their own conclusions.
In order to learn and draw conclusions, machines use an algorithm (i.e., a step-by-step guide) to complete a precise task, within a predefined framework, and to meet a specific need. To be a genuine source of understanding (and intelligence), this learning can only be achieved in partnership with humans—never by machines alone.
Ultimately, the true source of intelligence remains human. It is human intelligence that first understands the problem to be solved and designs the framework to do this. It is also human intelligence that is subsequently able to draw lessons from the learning process and the findings generated by the machine. In actual fact, artificial intelligence is really an extension of human intelligence rather than a form of intelligence in itself.
In short, AI technologies are a fantastic tool for assisting human intelligence. They pave the way for automation and faster decision-making, but human supervision is, and will always be, indispensable.
Machine learning is the core technology behind AI. It consists of teaching computers to autonomously find information within sets of data. Once a machine is given an example, it can sift through data, find patterns and correlations based on what it learned. When a machine starts drawing the right conclusions, it can then apply these learnings to new sets of data. The algorithm adapts and improves overtime as it processes more data.
Deep learning on the other hand, is a subset of machine learning. It mimics the neural network architecture of the human brain. Unlike machine learning, deep learning neural networks rely on several processing layers (that is where the “deep” comes from) to identify patterns, classify information or recognize speech, images, etc. Rather than relying on an example, deep learning algorithms process incredible quantities of raw data to learn and improve.
In recent years, deep learning algorithms have allowed AI technologies to penetrate countless markets and industries. Today, you would be hard pressed to find a sector—or even a person—that does not rely on AI solutions for some aspect of their business or everyday life. But what sparked this uptick? In large part, our own consumer habits have pushed deep learning to the forefront. Connected devices, smart cities, IoT in general, and our online habits—all these technological advancements produce an abundance of granular data from an incredibly diverse pool of sources. This large amount of data, paired with a marked increase in computer power, which began in the 1980s, more advanced algorithms and technology that became sufficiently mature in the early 2010s, ushered in a more efficient way of learning.
The ability to sort through massive amounts of data, brought by deep learning, exponentially boosts performance: problems can now be modeled with millions of parameters, deepening the learning process and providing answers to problems more complex than ever before. Carrying out tasks such as recognizing shapes or understanding speech has become amazingly efficient, boosting entire domains in the process.
Humans can often become overwhelmed by colossal volumes of data and, alone, are only able to exploit a finite portion—leaving large pools of data unused. Deep learning technology on the other hand is hugely scalable. By design, deep learning’s neural network becomes more efficient with the addition of new neurons. This means that machines can absorb a limitless amount of new data. Instead of reaching saturation, waves of data actually improve performance. As the network grows, performance increases and models become able to handle more and more complex problems. Deep learning is also an iterative process—meaning that it is a dynamic, self-actualizing system that is continuously adjusting to new data to find a better answer. This is yet another way that deep learning mimics the human mind—like us, deep learning algorithms improve with experience. But the comparison ends there.
Deep learning technology requires tons of data—the larger the data sheet, the better. But not just any data. Data collection needs to be precise and error free to ensure that the results are correct and unbiased. It also needs to be relevant, which means that data must be sourced without losing sight of the problem at hand. In other words, it is necessary to understand the applications in order to develop technological tools accordingly. The importance of data has skyrocketed in recent years—making it a true competitive advantage for those who source and use it properly. That last part is essential—data is, above all, a precious resource that must be safeguarded. That means that a clear framework concerning the ethics behind the ways in which data is gathered, stored and used is of utmost importance, especially considering how the use of data has evolved and will continue to evolve over the years.
While the concept of AI is inspired by the human mind, deep learning algorithms actually see patterns more easily than we do, thus easing the decision making process and facilitating automation without fatigue. AI is able to both handle tasks that are beyond the scope of traditional computers, while also completing time-consuming, tedious tasks faster and more consistently than humans. Concretely, AI solutions helps improve prediction, detection and sorting across all domains—for example, object detection and recognition—thereby freeing up precious time that can then be spent on added-value tasks that require human intelligence.
The advantages of AI technologies are unmistakable when it comes to analyzing large pools of data. From analyzing routes and identifying patterns to boost efficiency and the user experience in the transportation industry; sifting through wearable device data to improve patient care and diagnosis in the medical field; or personalizing the marketing and shopping experience in the retail sector—innovative AI use cases are expanding across industries and sectors. Financial institutions and government agencies also rely on AI solutions to detect fraud and protect citizens and businesses. Backed by AI-generated insights, decision makers are better equipped to make the right call.
There is a lot of talk about bias in biometrics. Afterall, in the scientific community, there is an entire field of research, “fairness in machine learning systems,” dedicated to measuring, understanding and attenuating issues of bias. But in reality, bias is far more common in human nature and in society at large than in balanced and accurate algorithms. In fact, today’s algorithms present a viable solution to this real world problem. That being said, it is essential to remember that AI solutions must always be combined with human analysis and decision. In the case of law enforcement, justice, or border authorities using AI technologies, the final decision always rests with the people who are sworn in and authorized by law to make the call. AI technologies are only a support for the human to accomplish a task, speed it up, while reducing the risk of error.
The identity and security industry has come a long way in reducing bias in biometric recognition. Notably, over the past few years, independent test conducted by the National Institute of Standards and Technology (NIST) have shown that the differences in performance of biometric identification algorithms between demographic groups can be reduced to a point that they are virtually undetectable. Best-in-class AI facial recognition technology stands out in these tests by combining fairness and accuracy.
It is important to note that to achieve that balance these algorithms do not learn by themselves once deployed—humans keep control over their performance. Developers measure error rates of the algorithms on different groups, apply corrective measures and ensure fairness remains a key criteria.
With the ability to identify all subjects equally well, regardless of demographic, AI technologies developed in a responsible and ethical way can actually help humans reduce the risk of discrimination.
Advancements in AI have revolutionized the field of biometrics. The ability to model more complex problems and process more data much faster have raised the bar substantially in terms of performance and accuracy. For starters, the sheer amount of data available combined with the computing capabilities brought about by deep learning make biometric algorithms more accurate than ever before. While facial recognition is perhaps the best example of the impact AI has on biometrics, deep learning has also driven advancements in fingerprint technology, and is just starting to scratch the surface in the realm of iris technology as well.
In the early days of facial recognition algorithms, it was only possible to identify a face when positioned directly in front of a biometric terminal. Progress in this field, mainly driven by AI increasing efficiency, has improved the user experience.
Today’s AI facial recognition technology requires very little of the user while their identity is verified—the process is faster, more efficient and frictionless. For example, a user’s face can be accurately analyzed whether they are moving or static, wearing glasses or smiling, facing the biometric terminal or looking in another direction. AI algorithms can even achieve liveness detection without asking the subject to perform any specific pose or movement. Liveness detection—or the ability to confirm that the analyzed face or fingerprint is, in fact, actually presented, in person, by their real owner (versus a photo, silicone mask or a spoofed fingerprint)—drastically improves anti-fraud systems.
When it comes to fingerprint biometrics, deep learning technology makes it possible to read even damaged fingerprints or accurately verify identity via a fully contactless access control system.
Today’s access control systems can also rely on facial biometric data to identify visitors and employees from a distance as they enter a building. Advanced algorithms can create a truly seamless biometric identification experience by enabling in-motion recognition while ensuring the highest accuracy. The strength behind this technology resides in the ability of AI algorithms to analyze the entire situation around access points, enabling group access and detecting suspicious behaviors simultaneously.
And as the world in general veers more and more towards contactless methods, so, too, does the field of access control. Quite simply, using an AI facial recognition system means that no direct contact is needed with an access control equipment, a much more hygienic alternative in a post-pandemic climate.
Another real world example of deep learning technology at work is the verification of a vast array of documents—including passports, driver’s licenses, visas, immigration papers, tax documents, voter identification cards and more. Deep learning algorithms can detect documents placed on a scanner or in front of a phone camera, identify the type of document, read the text and images, and ensure the authenticity—verifying that it is not a false document or a photocopy, for example. This means analyzing fonts, security features such as holograms and watermarks and bar codes, and being able to identify image manipulation, pixel tampering, digital tampering and other types of forgeries. Here, AI is an invaluable resource—simultaneously verifying all the security features of a document more efficiently, more rapidly and more securely than ever before. AI is being able to achieve all that on a multitude of documents, even remotely—a task on which even the most trained human mind cannot compete.
Whether you are aware of it or not, AI is at work in various parts of everyday life—for companies, governments and end-users alike. By advancing biometrics, image analysis and anti-fraud systems, AI solutions help protect identities, streamline their verification, and make the world run just a little bit smoother.
AI is present in every advanced type of identity verification and fraud detection situation—both in-person and online:
One specific example of deep learning at work in a decision making process is in the context of passenger flow facilitation. Here, a whole chain of AI algorithms is required at all stages of an automated identity verification process. The first step is detection and tracking. For example, understanding all the elements in an eGate video feed or locating the iris on a person’s face. Next is quality assessment, finding the best images to use for biometric purpose. Then building a biometric template, i.e extracting relevant information from the image. Last is recognition, or matching similar data. In this example, deep learning algorithms confirm a passenger’s identity when they scan their passport upon check-in and when they step in front of a camera at an eGate for a final biometric check before boarding.
In sum, AI compares a passport image (and verify that the photo has not been tampered with) with the live image to determine that the person is who they say they are—all within in a few seconds.
While the legal framework concerning the use of AI to ensure public safety raises legitimate ethical questions around the word—and will continue to evolve, AI solutions have been and will continue to be incredibly useful in very precise situations. First, in identifying victims of crimes; second, in searching for people convicted or suspected of serious offenses; and lastly, in the event of a serious or immediate public safety threat. In these situations, AI can be used to automatically extract faces or vehicles or other objects that appear in video footage and send automated alerts when found. It makes sense of all available data; saves time, resources and money—all while reducing human error.
Technology isn’t good or bad in itself, it’s all about how you apply it. In the identity and security industry—where people use machines to verify identities by relying on biometric data, for instance—ethics is an extremely important factor. In order to alleviate legitimate public concerns and create trust, the industry must be vigilant and hold itself accountable when it comes to the application of these groundbreaking technologies. Strict do’s and don’ts must be applied. For example, human supervision is essential. While AI technologies have allowed the industry to advance by leaps and bounds, we can’t forget that there is a real difference between a machine and a human. Governments and third parties have also stepped in to create regulatory frameworks to ensure the responsible use of technology.
Implementing an industrial process with tests and validations, every step of the way, is an important part of developing technologies that rely on deep learning algorithms. For example, the way that machines learn must always be supervised by a human being. And while improving performance is important, it is crucial to identify and correct potential bias—a very complex task, but nonetheless a major part of the job.
In recent years there has been a marked shift in the way performance is measured—notably, the National Institute of Standards and Technology (NIST) includes bias correction control in its facial biometrics benchmark evaluations since 20191. This means that bias correction is not only possible, but it is also a qualitative criteria when it comes to assessing the performance of an AI facial recognition algorithm. After all, data creates a huge advantage, but it has its limits. If the dataset is not representative enough, for example, it can lead to bias. This happened in the early days of deep learning (some initial deep learning experiments fell into the trap of gender or ethnic biases). These mistakes have since been corrected. Today, a database can be analyzed through statistics, allowing it to detect a potential imbalance in the dataset and then counterbalance it.
Another example of human intervention was when programmers and engineers realized the absolute importance of obtaining a high-quality image of all skin tones in every lightning condition. In order to avoid bias. With the help of AI they created a control loop to optimize the gain and adjusted the camera shutter to guarantee the same image quality regardless of skin color. Another way to eliminate bias is to work directly on the number of images and identities per group in the learning dataset.
With growing concerns around privacy protection, it is important to note that responsible actors in the field are committed to protecting end-user privacy by only using anonymized databases to train AI algorithms. From a technological standpoint, when training an algorithm to recognize faces there is no need to attribute biometric data to a specific person— the algorithm only needs pictures of the same face from different angles, in various lighting conditions, with various accessories or haircuts, or at different ages. In other words, users only need answers from the system, not the actual data used by the AI algorithms in the decision-making process.
To quell any lingering concerns, authorities and privacy regulations such as GDPR in Europe, have been set up to define clear guidelines for the collection and use of data, and to ensure compliance.
With the technology wave continuing to swell, it’s safe to say that data volumes will only continue to grow exponentially. That means that there is a sizable amount of unlabeled data being created every second of every day—data that is not yet being used to its full potential. This shift in data volumes will most certainly continue to power AI models and use cases in the future.
The shift on the horizon is the move from supervised (using labeled data only) to semi-supervised learning (using labelled data and unlabeled data), weakly-supervised (using indirect labels) or even unsupervised learning (using only unlabeled data). These technics allow to increase data usage even when labels are unavailable or too difficult to produce. But let’s be clear, whether the data is labeled or not, the learning process – and the measurement of its performances – will remain under human supervision.
We are starting to understand what goes on inside neural networks; a process that will continue to advance in the years to come. Today, AI experts are looking further into how deep learning algorithms reach the conclusions that they do, particularly when they do not reach the expected result. That said, it is important to remember that while an algorithm allows a machine to learn on its own, it is also backed by an industrial process. Humans still have the extremely important task of validating, testing, measuring results and doing whatever it takes to ensure algorithm accuracy. We humans cannot simply develop the technology and “let it loose.”
At IDEMIA, AI is not just a tool to analyze business data or optimize logistics as in many other companies, it is at the core of the solutions we develop. More precisely, we use it to enable our systems to derive meaningful information from visual inputs, and act or make recommendations accordingly.
First and foremost, IDEMIA recognizes the sensitive nature of all personal. We are committed to data protection, not only when training AI algorithms and developing solutions but also when our solutions are used in the field. We consider it absolutely essential to make sure that our solutions cannot be hijacked, tampered with or circumvented. To this end, we pay special attention to how data is handled and to the regulations that apply. In fact, long before GDPR regulation existed, we had set up our own processes and infrastructure to securely manage personal data—these processes are now also in compliance with privacy regulations such as GDPR in Europe, USA privacy regulations or their equivalent in other regions.
In order to create the most accurate algorithms, we constantly need to access more data—responsibly. We obtain data from clients, in compliance with relevant privacy regulations, to train their algorithms and provide high-performance products and solutions. We also rely on data shared on a voluntary basis by our employees to build up our database year after year. Lastly, we create synthetic images using a Generative Adversarial Network (GAN). This enables us to generate qualitative synthetic facial images and fingerprints that are completely fictional. So, when a client asks us to share data to test the efficiency of our algorithms, we can share our synthetic data.
As a leader in our field, we are committed to create solutions that protect personal data and ensure it cannot and will not be misused. To do so, we apply our expertise in cryptography techniques and access management rights to design databases in a way that allows authorized users to search a database for a particular person without giving them access to the list of people in that database. That means that no one can extract personal data—not IDEMIA, nor our clients, governments or anyone who may attempt to break in. Furthermore, whenever this is possible, we design solutions and systems to ensure that personal data is held only by their individual owners—encrypted in the secure element of a document, a smartcard or smartphone, for example.
As technology and cryptographic techniques continue to advance, we keep investing in new ways to further protect personal data and ensure restricted access to such data.
Today, we can proudly say that our deep learning algorithms are so efficient that biases can hardly be measured—a claim that is equally bold and rare in our industry. But this is no small task! Our dedicated teams are experts in the complex process of preparing training data and adjusting the way an algorithm learns on a given data set. They also ensure that our databases contain a variety of images of the same element in various acquisition conditions to guarantee true inclusiveness.
Everyone in the ecosystem has to do their part to protect users. This includes industrial players like IDEMIA, national and international working groups and think tanks, academics, regulators and clients using the technology. Over the years, IDEMIA has positioned itself as a privileged partner in the French AI ecosystem. We work closely with the CNIL and the French National Research Agency; participate in workshops hosted by The World Economic Forum’s Facial Recognition Project; support the academic ecosystem by working with several high-level research chairs, notably on AI. Moreover, we make it a point to carefully analyze how our customers might use our AI solutions and partner only with those who align with our ethical standards.
In an increasingly competitive international context, we call for strict regulations around data collection for research purposes in order to comply with ethical standards, while also supporting industry competitiveness. Moving forward, we plan to continue to explore other avenues as well—such as the creation of a label for “trusted suppliers” at the European level for example. When we consider that AI facial recognition can be used in various government contexts (border control, for example.), guaranteeing the origin of the technology is not only a key element of national sovereignty, but it raises questions about performance, methodology, ethics and more. We consider it imperative to have a structure that can help customers calmly choose the technological solution that best suits their needs, based on clearly defined and evaluated technical criteria. The end goal is that someday, customers using “trusted” AI—or more precisely the resulting technology—can be sure it abides by all industry standards.
1 https://www.nist.gov/publications/face-recognition-vendor-test-part-3-demographic-effects
Latest News